The elusive video search whereby you can search video image context is now possible with advanced technologies like deep learning. It’s very exciting to see video SEO becoming a reality thanks to amazing algorithms and massive computing power. We truly can say a picture is worth 1,000 words!
Content creators have fantasized about doing video search. For many years,, major engineering challenges were a road block to comprehending video images directly.
Video visual search opens up a whole new field where video is the new HTML. And, the new visual SEO is what’s in the image. We’re in exciting times with new companies dedicated to video visual search. In a previous post, Video Machine Learning: A Content Marketing Revolution, we demonstrated image analysis within video to improve video performance. After one year, we’re now embarking on video visual search via deep learning.
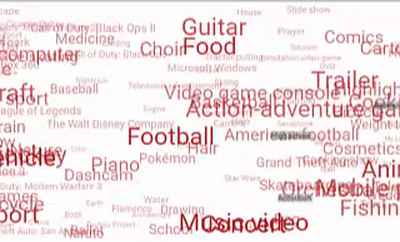
Behind the Deep Curtain
Many research groups have collaborated to push the field of deep learning forward. Using an advanced image labeling repository like ImageNet has elevated the deep learning field. The ability to take video and identify what’s in the video frames and apply description opens up huge visual keywords.
What is deep learning? It is probably the biggest buzzword around along with AI (Artificial Intelligence). Deep Learning came from advanced math on large data set processing, similar to the way the human brain works. The human brain is made of up tons of neurons and we have long attempted to mimic how these neurons work. Previously, only humans and a few other animals had the ability to do what machines can now do. This is a game changer.
The evolution of what’s call a Convolution Neural Network, or CNN aka deep learning, was created from thought leaders like Yann LeCrun (Facebook), Geoffrey Hinton (Google), Andrew Ng (Baidu) and Li Fei-Fei (Director of the Stanford AI Lab and creator of ImageNet). Now the field has exploded and all major companies have open sourced their deep learning platforms for running Convolution Neural Networks in various forms. In an interview with New York Times, Fei-Fei said “I consider the pixel data in images and video to be the dark matter of the Internet. We are now starting to illuminate it.” That was back in 2014. For more on the history of machine learning, see the post by Roger Parloff at Fortune.
Big Numbers
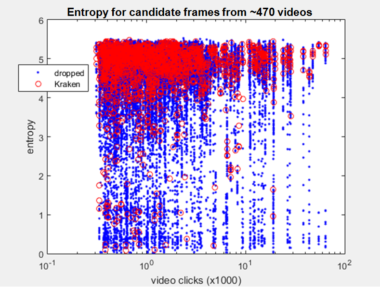
Think about this: video is a collection of images linked together and played back at 30 frames-a-second. Analyzing massive number of frames is a major challenge
As humans, we see video all the time and our brains are processing those images in real-time. Getting a machine to do this very task at scale is not trivial. Machines processing images is an amazing feat and doing this task in real-time video is even harder. You must decipher shapes, symbols, objects, and meaning. For robotics and self-driving cars this is the holy grail.
To create a video image classification system required a slightly different approach. You must handle the enormous number of single frames in a video file first to understand what’s in the images.
Visual Search
On September 28th, 2016, the seven-member Google research team announced YouTube-8M leveraging state-of-the-art deep learning models. YouTube-8M, consists of 8 million YouTube videos, equivalent to 500K hours of video, all labeled and there are 4800 Knowledge Graph entities. This is a big deal for the video deep learning space. YouTube-8M’s scale required some pre-processing on images to pull frame level features first. The team used Inception-V3 image annotation model trained on ImageNet. What’s makes this such a great thing is we now have access to a very large video labeling system and Google did massive heavy lifting to create 8M.

The secret to handling all this big data was reducing the number of frames to be processed. The key is extracting frame level features from 1 frame-per-second creating a manageable data set. This resulted in 1.9 billion video frames enabling a reasonable handling of data. With this size you can train a TensorFlow model on a single Graphic Process Unit (GPU) in 1 day! In comparison, the 8M would have required a petabyte of video storage and 24 CPUs of computing power for a year. It’s easy to see why pre-processing was required to do video image analysis and frame segmenting created a manageable data set.
Big Deep Learning Opportunity
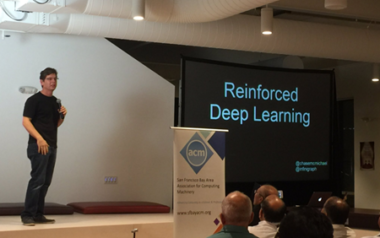
Google has beautifully created two big parts of the video deep learning trifecta. First, they opened up a video based labeling system (YouTube8m). This will give all in the industry a leg up in analyzing video. Without a labeling system like ImageNet, you would have to do the insane visual analysis on your own. Second, Google opened Tensoflow, their deep learning platform, creating a perfect storm for video deep learning to take off. This is why some call it an artificial intelligence renaissance. Third, we have access to a big data pipeline. For Google this is easy, as they have YouTube. Companies that are creating large amounts of video or user-generated videos will greatly benefit.
The deep learning code and hardware are becoming democratized, and its all about the visual pipeline. Having access to a robust data pipeline is the differentiation. Companies that have the data pipeline will create a competitive advantage from this trifecta.
Big Start
Follow Google’s lead with TensorFlow, Facebook launched it’s own open AI platform FAIR, followed by Baidu. What does this all mean? The visual information disruption is in full motion. We’re in a unique time where machines can see and think. This is the next wave of computing. Video SEO powered by deep learning is on track to be what keywords are to HTML.
Visual search is driving opportunity and lowering technology costs to propel innovation. Video discovery is not bound by what’s in a video description (meta layer). The use cases around deep learning include medical image processing to self-flying drones, and that is just a start.
Deep learning will have a profound impact our daily lives in ways we never imagined.
Both Instagram and Snapchat are using sticker overlays based on facial recognition and Google Photo sort your photos better than any app out there. Now we’re seeing purchases linked with object recognition at Houzz leveraging product identification powered by deep learning. The future is bright for deep learning and content creation. Very soon we’ll be seeing artificial intelligence producing and editing video.
How do you see video visual search benefiting you, and what exciting use cases can you imagine?
Feature Image is YouTube 8M web interface screen shot taken by Chase McMichael on September 30th .