This is part 1 of 2 in a series of articles where I will layout a vision to bring more objectivity to SEO by applying a search engine engineer’s perspective to the field. I will present a step-by-step way to statistically model any search engine. This method can be, among many things, used to reveal specific courses of action that marketers can take to intelligently improve organic click-through rates and rankings for any given set of websites and keywords.
Full Disclosure: I am the Co-Founder and CTO of MarketBrew, a company that develops and hosts a SaaS-based commercial search engine model.
The Times, They Are a Changin’
It’s been almost 17 years now since Google was born, and the search engine optimization (SEO) industry, an industry that has been fervently dedicated to understanding and profiting from search engines, is finally catching up to the (technical) nature of search.
Search, on the other hand, has always been a highly technical space, filled with thousands of Ph.D’s in Computer Science solving incredible problems with their rapidly evolving algorithms. SEO, for the better part of those 17 years, was filled with non-technical marketers, and to date has mostly been an art form.
This wasn’t a coincidence – for most of the elite engineers coming out of Stanford, M.I.T., Carnegie Mellon, and others, the money was in building search engines, not understanding them.
But as the famous songwriter Bob Dylan once said, the times they are a changin’. In 2015, digital marketing is growing at a rapid clip and pay-per-click (PPC) is becoming over-saturated and highly competitive. For many companies, this means PPC is no longer a profitable marketing channel. In addition, CMOs are finally starting to turn to the science of SEO to help them quantify the risk associated with things like content marketing and organic brand promotion.
Search engines have had a direct hand in this of course: their continued stance of taking away feedback for their organic listings has led many in the SEO profession to switch to PPC or redefine SEO altogether.
SEO: A Law of Nature?
The technology approach that I am about to propose is already available in every other marketing channel known to man. In fact, the availability of advertising metrics has always been a requirement of any legitimate marketing campaign – if you cannot measure your marketing, then how can you define ROI?
SEO, or the business of improving your brand’s organic visibility on search engines, has always been viewed as a marketing channel by everyone, except search engines. Why? Search engines are highly dependent on advertising revenue, which depends on not knowing how organic search works. In fact, for all of the amazing things that Google is involved with today, revenue from PPC for Google in 2013 alone made up 50 out of its 55 billion in total revenue.
While Google may not share the industry’s view that organic search is a marketing channel, it does not change the fact that it is. And although Google owns a majority of the search market as of this writing, this ideology is completely agnostic to Google. What I am about to share with you will work with any search engine, for it is a law of the nature of search.
A (Customizable) Search Engine Model
Algorithmic Modeling
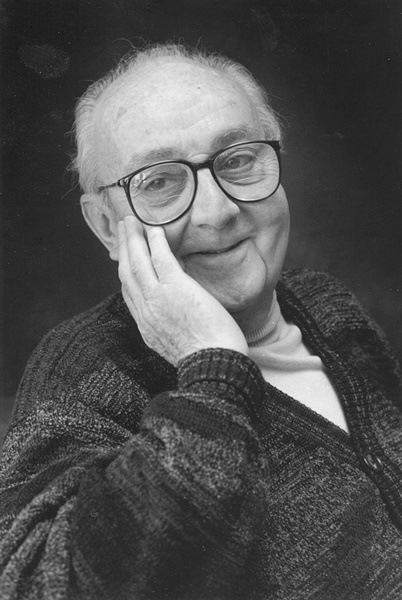
As the famous statistician George E.P. Box (pictured right) once said, “All models are wrong, but some are useful.” No search engine model will ever truly replicate the actual search engine being modeled. But, you don’t need to perfectly replicate a search engine to get utility out of this approach. You simply need something that has a positive correlation.
Elon Musk is famous for solving very complex processes by reasoning from first principles. I propose the same approach here. The first step in developing a predictive model for a search engine, in this case, is to develop and model a family of algorithms that make up the core principles of any search engine. I define “core algorithms” to mean those that have been around for at least a few years and which are likely to stick around in the future.
Keep in mind, as you get more specific/narrow with your algorithms, your model will become more fragile. This is because the more specific your algorithm is, the more likely that this algorithm can change in the future. Core algorithms, like the ones shown here, have been around for years, and continue to be the bedrock of any search engine.
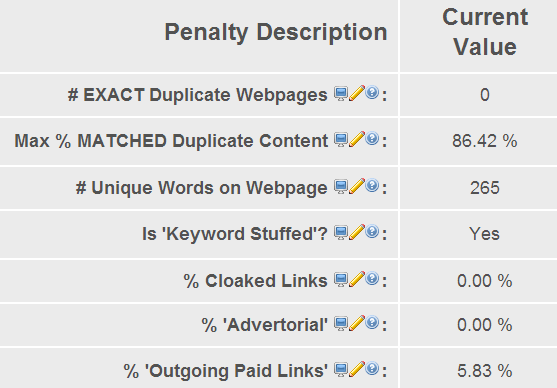
The trick here is to make your model customizable: if you have the ability to assign different weights to each algorithmic family, you will be able to adjust these in the future as you begin testing your correlations with reality.
Off-Page Modeling
Another incredibly important piece of this search engine model is off-page or backlink data. Calculating PageRank is not enough: each link should be modeled with its own core set of algorithms, much like a modern PageRank algorithm would do.
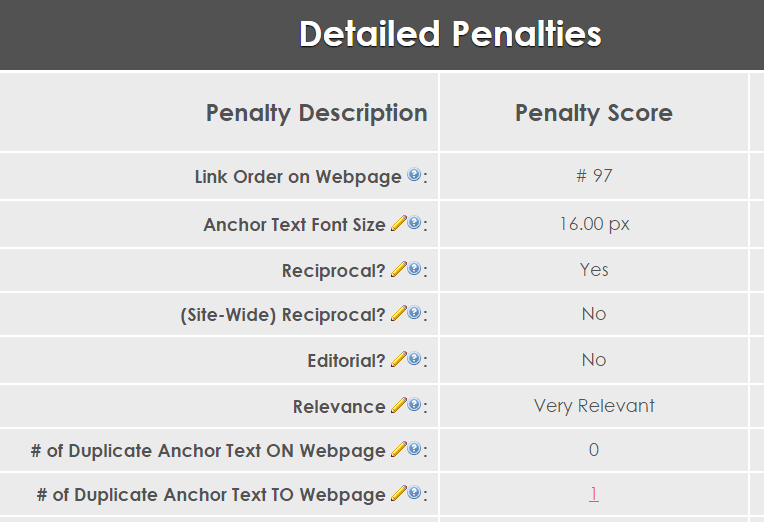
Link Graphs today are becoming a commodity, but the best ones operate in context of a Search Engine, scoring content in addition to links, all part of the backlink curating process.
Transparent Search Results
The final component of the search engine model is the ability to show the query scoring distances between ranking #1, #2, #3, and so on…for any given keyword.
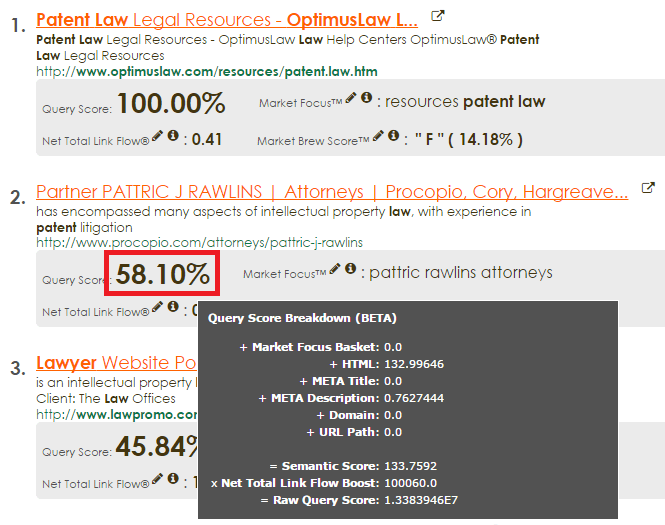
This is the feedback step in the model – the step that makes all of this possible – it gives us the ability to see if our algorithmic models are correlating with reality, so we can adjust if necessary.
Again, each of the query score sub-components should be customizable; for instance, if we see our model is too heavily weighting the meta title, we should be able to dial that back. If we think the off-page component is not factoring into the results enough, then we can dial that up.
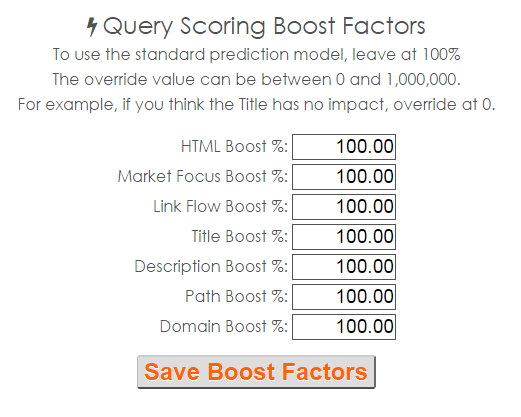
Once we have put this together, we then have the unique ability to see search ranking distances, which you can then use to do some very interesting things, like evaluating the cost/benefit of focusing on specific scenarios. I will get into this and more in the second part in this two-part journey.
The Self-Calibrating Search Engine Model
One of the most familiar ways to measure a positive correlation is called Pearson’s correlation coefficient, a fancy mathematician’s formula that tells us how close we are to modeling the true signal. We take what we see in reality, use similar algorithms to model what we think is happening, and then compare the two.
Throughout building this search engine model, we will want to correlate what we see in the model with reality, using a metric similar to Pearson’s correlation coefficient.
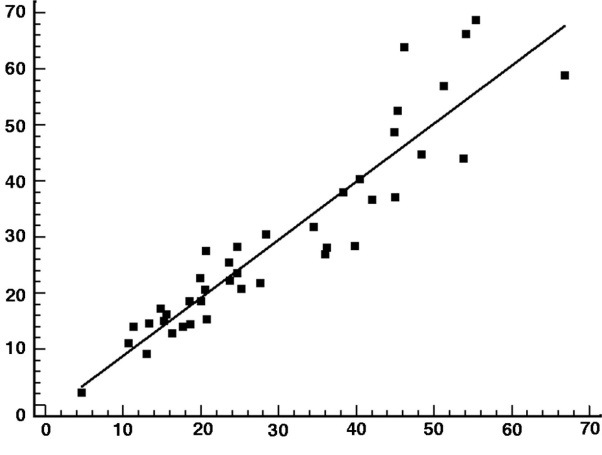
A self-calibrating search engine model can then be constructed by sweeping each query score factor through its range of weights, across hundreds of keywords and websites, comparing it to the target search engine environment, and then pick the highest correlated setting automatically. Think of this as a self-calibration feature.
Next Steps
Once we have a positively correlated search engine model, we can do amazing things!
Some examples of questions this approach can solve:
- How far do I have to go to pass my competitor for a specific keyword?
- When will my competitor pass me in ranking for a specific keyword?
- Resource planning: given 1,000 keywords, which ranking scenarios will cause the biggest shift in traffic?
In addition, this system can also predict search results months in advance of traditional ranking systems, because traditional ranking systems are based on month’s old scoring data from their respective search engine.
In a future article, I will discuss the second part of this vision: how we can use this model to reveal exciting opportunities in specific competitive markets, predict when you will pass your competition (or when they will pass you!), and potentially change the way we view and implement SEO altogether.
It’s 2015, search is all grown up, and those who choose to embrace this technical approach will find that SEO is no longer about some black-hat magic trick or the latest algorithmic loophole. It isn’t about trying to subvert existing algorithms by tricking dumb search engines.
Organic search should be treated like any other marketing channel – a clearly defined risk versus reward environment that gives CMOs useful metrics that help them determine the viability of their SEO campaigns.
Image Credits
Featured Image: yanugkelid via Shutterstock
Image #1: Box image via Wikimedia Commons
All screenshots taken from Market Brew patented system in 2015.